AI & AIoT
Five Minutes to Know More About the Deep Learning Industry – Will AI Replace Humans?
Can Artificial Intelligence really mimic a human being? And what are the most popular examples of deep learning applications in recent years?

Can Artificial Intelligence Really
Mimic a Human Being?
What Are Some Popular Examples of Deep Learning Applications in Recent Years?
Image Recognition Technology
Natural Language Processing
Recommender Systems
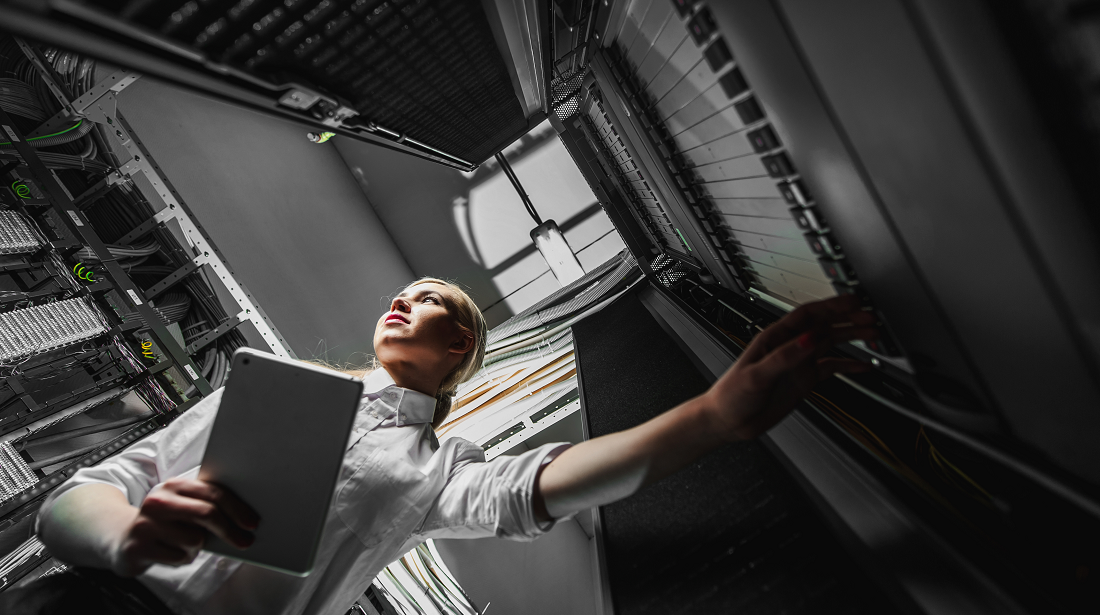
The Challenges with Developing Deep Learning Technologies
Deep Learning Hardware & Software Architecture
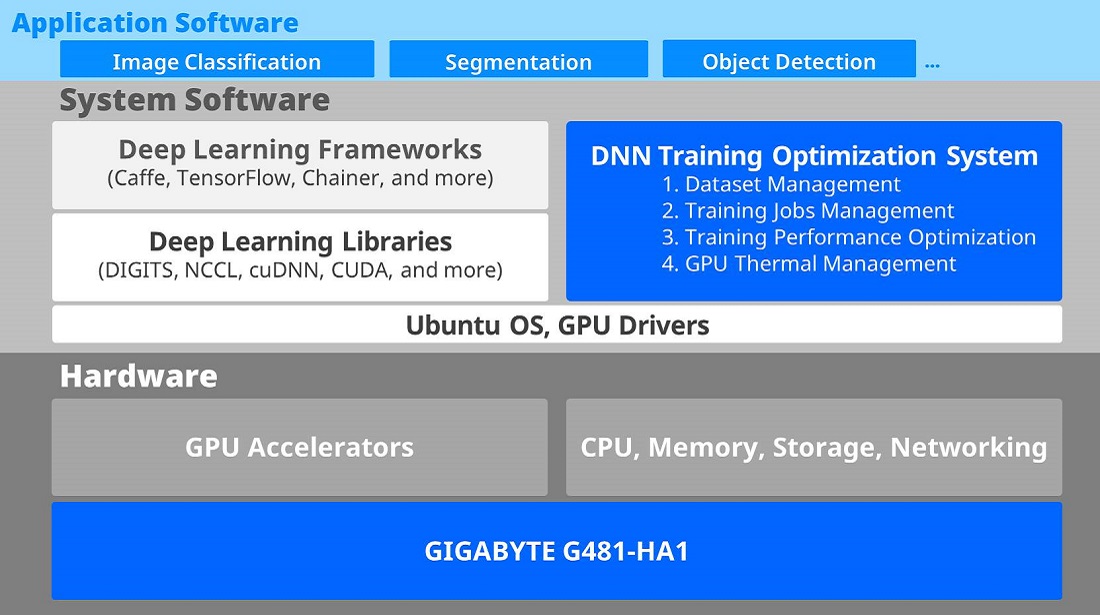
GIGABYTE’s DNN Training Appliance Provides a User Friendly Development Environment
Mature Deep Learning Solutions Will Allow Major Companies to Quickly Implement Applications
Get the inside scoop on the latest tech trends, subscribe today!
Get Updates
Recommended Reading
# Artificial Intelligence (AI)
# Machine learning (ML)
# Natural Language Processing (NLP)
# Deep Learning (DL)
# Cloud Computing
# 5G
# AI Training
# AI Inference
# Generative AI (GenAI)
# Digital Twin
# Computer Vision
Get the inside scoop on the latest tech trends, subscribe today!
Get Updates